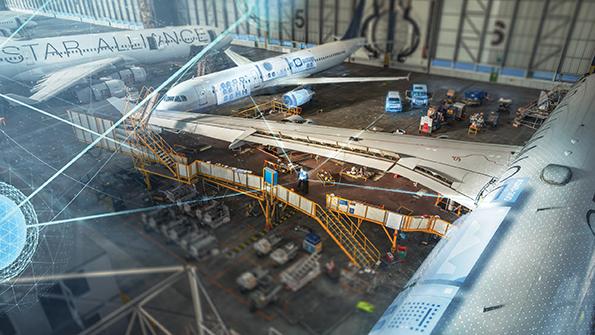
As the aviation industry builds momentum toward a full recovery, one of the key bottlenecks is finding replacements for workers lost to downsizing during the pandemic.
Skilled engineering personnel are in particularly short supply, as many have left for other sectors.
This is an acute problem for manufacturers, airlines and aftermarket providers, although it is also one that can be ameliorated in certain areas through digitalization, which can free skilled workers from some repetitive, labor-intensive tasks.
Automated damage recognition is one example of where technology can help; another is predictive maintenance to avoid unscheduled removals and increase time on wing.
“The pandemic was a key driver for many airlines in the world to invest in digital solutions, which will enable them to become more efficient and lean going forward with less staff or the same staff with a growing fleet,” Mia Witzig, head of Lufthansa Technik’s “digital solutions: predict & recommend,” tells Inside MRO.
In response, Lufthansa Technik (LHT) has continued to invest in digital products throughout the crisis, consolidating new guidance in a new Technical Logbook, new MRO management systems and many new predictors for Airbus and Boeing aircraft.
The effort was rewarded in early 2021, when United Airlines agreed to work with LHT to develop predictive maintenance systems for the more than 600 Boeing 737 aircraft in the U.S. carrier’s fleet, a huge source of data that LHT said would produce “unique” predictive maintenance systems for United.
Predicted Recovery
Lee Hui Fung, senior vice president and head of innovation and continuous improvement for commercial aero-space at ST Engineering, notes that industry recovery has spurred interest in predictive maintenance.
“As international borders began open-ing and aviation activities started to ramp up in the beginning of the year, we have been seeing more airline customers who want to incorporate predictive maintenance into their renewed or new maintenance-by-the-hour contracts with us for their critical aircraft components,” she says.
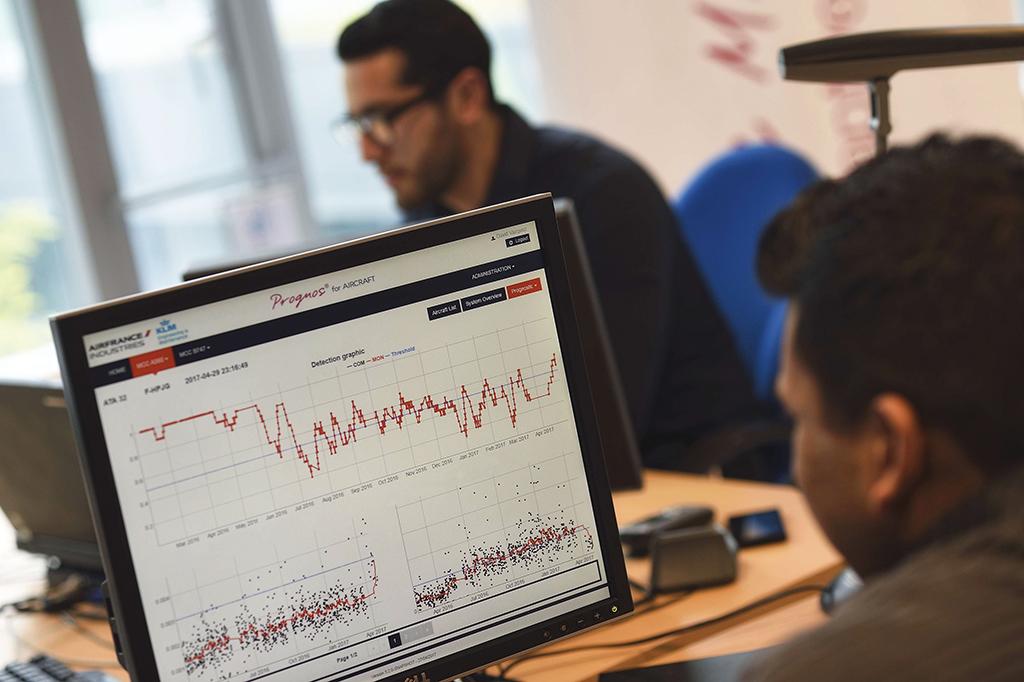
This may accelerate penetration of predictive technology, which—outside of engine applications—was struggling to gain traction with many airlines prior to the pandemic. In 2021, LHT estimated that only about 50 airlines were using true predictive maintenance—where predictions led to maintenance actions—for non-engine components. Airbus, meanwhile, said at the time that about 10% of the global A320 fleet was covered by the OEM’s predictive maintenance program.
Data sharing has been a major hurdle for adoption of the new technology, with many airlines either unable or unwilling to share the data that enables predictive algorithms. As such information became increasingly valuable, OEMs and operators guarded it more jealously. Sometimes this meant airlines could not access the operational data they needed, while even when they had it, some feared that sharing such data with third-party MRO providers would benefit competitors as much as it would themselves.
“If customers are not ready to change yet, they are very often not ready to deliver the right data in the required quality, which consequently leads to less valuable results. It is therefore key that the leadership team supports the process of digitalization, selects the right people and partners,” says Witzig.
Technology can also help, says Rik van Lieshout, digital products and services manager for Air France Industries KLM Engineering & Maintenance. “Running machine learning algorithms on multiple datasets, for example, distributed over multiple operators, would enable algorithm development without direct access to another operator’s data.”
To adopt predictive maintenance in full, airlines must also be willing to remove serviceable components that algorithms suggest-—otherwise they risk incurring greater cost or downtime later.
Witzig says that customers “need to be open to innovative solutions and be prepared to change their traditional processes if required and valuable.”
“It requires collaboration between companies to value a prediction; only afterward can you tell if the action was valid,” adds van Lieshout. “As the supplier of Prognos [Air France-KLM E&M’s predictive maintenance tool], we have the luck to work with our own Air France-KLM fleet, so we can show those who need to be convinced our own experiences where we clearly see the value.”
Use Cases Prove The Value
LHT offers predictive maintenance via its Aviatar platform, which it says helps up to 30% of unscheduled removals.
The MRO provider has developed many new predictors during the crisis, including for the bleed and pneumatic systems on A320neo and 737 APU and hydraulic systems. In one instance, LHT says it saved about $4,000 in annual expenses linked to the bleed system pressure regulator valve, with savings coming from avoiding troubleshooting labor and saving about 300 kg (660 lb.) of fuel for an engine-ramp-up.
Looking ahead and in response to input from customers, LHT would like to extend predictive systems to the Boeing 787 cabin air compressor and the A320ceo flow control valve.
AFI KLM E&M’s Prognos for Aircraft takes continuous aircraft sensor data to calculate health metrics of various components each flight. The algorithms that calculate the health metrics are developed using a large pool of historical operational data in combination with artificial intelligence. If, on live data, the system recognizes unhealthy behavior, an alert is triggered and sent via e-mail. The end user can analyze the situation through a graphical user interface and plan proactive maintenance actions accordingly.
One of the functions of its current product is to track the health of fuel tank sensors, malfunction of more than one of which can lead to extended aircraft-on-ground times as engineers must enter the tank to replace the sensors.
“Since we use Prognos, we monitor the health of each individual sensor, so a single failure is noticed before it impacts aircraft operation. By replacing these sensors proactively at every convenient maintenance opportunity, we have saved a lot of unplanned ground time of our fleet,“ says van Lieshout.
Prognos has also helped optimize inventory planning and troubleshooting times for cargo cooling systems, he says, allowing AFI KLM E&M to predict system failures and order replacement parts well ahead of any adverse event.
ST Engineering offers predictive maintenance through its in-house component management product. It integrates “Component Health Management” with other component services such as parts requests and fleet component reliability analysis. As more customers adopt predictive maintenance, it plans to integrate engine health management and airframe MRO systems into Maestro, its one-stop portal for MRO services.
“Our customers collaborate with us by providing about two years of relevant historic sensor flight data, which, in combination with MRO information on the selected components, enables us to develop a proof of concept that yields acceptable accuracy in predictive maintenance,” says Lee.
New Versus Legacy Aircraft
Tapping into operational data to develop and refine algorithms is a key function of predictive maintenance, so it might seem that older aircraft types, with more accumulated flight hours, can benefit the most from the technology. However, the latest generation of aircraft makes up for short operational histories with bigger sensor and communications suites, which generate far more data per flight than older models.
“The extensive maintenance experience and historical data on legacy aircraft types is definitely advantageous for developing algorithms,” says van Lieshout. “On the other hand, newer- generation aircraft tend to have more onboard sensors and better connectivity, so the missing [duration] of the data is made up by wider data in terms of parameter scope.”
Witzig agrees, noting that “from an engineering perspective, newer aircraft types are more interesting for developing new prediction use cases, as they are talking a lot more than the old ones.”
One example of this is to develop digital twins of systems and components. A full-flight data stream of an A350, for example, allows LHT to rebuild the behavior of components during different flight phases.
“That helps us enormously while trying to predict this behavior—and the possible failure,” says Witzig. “The troubleshooting starts more or less already in the air and once the aircraft comes to the shop on ground, Aviatar gives the airline all the information about the root cause and what maintenance action has to be performed.”