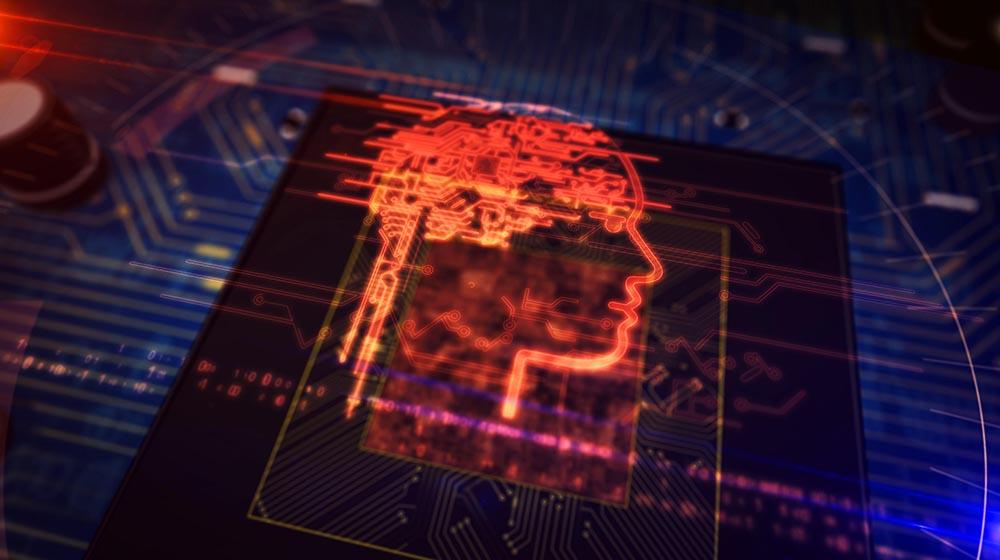
The new generative artificial intelligence (Gen-AI) tools are trained on the patterns and structure of input data and support applications such as ChatGPT. How close is Gen-AI to helping aircraft maintenance?
When Aviation Week raised this question with AFI KLM E&M, it received an answer from Talia, the private Gen-AI app of the Air France-KLM Group, speaking on behalf of IT Innovation Manager Jeroen Mulder and Group Innovation Director Ahmoss Messayeh.
Speaking through Talia, Messayeh says that in the short-term, Gen-AI will help with predictive maintenance. “Gen-AI can analyze data from sensors and other sources and learn from historical failure patterns to predict when maintenance is required, reducing downtime and maintenance costs,” he says.
Also in the short term, Gen-AI will help customer service. “Gen-AI can be trained to help our employees give fast and accurate answers to some time-sensitive requests,” Messayeh argues. But human interaction will remain crucial, and Gen-AI will only be supportive.
Longer term, Messayeh sees Gen-AI helping with more complex tasks such as supply chain optimization. “Gen-AI can analyze data from suppliers, logistics providers and customers to help optimize the supply chain, reducing costs and improving efficiency,” he says.
The new tool will eventually aid risk management, analyzing risks, spotting potential threats and helping to mitigate risks. “Overall, Gen-AI has the potential to transform various business functions, providing businesses with a competitive advantage and improving their bottom line,” Messayeh says.
However, Innovation Manager Mulder highlights some of the hurdles to exploiting Gen-AI.
These include ensuring that the data used to train Gen-AI is accurate, complete and relevant. Skills will also be critical, and the MRO may need to hire or train new experts, such as prompt engineers, and educate all potential users to make sure they question Gen-AI results.
Mulder is also concerned with security and privacy, ensuring data is secure and usage complies with data protection and ethical regulations. “This requires both technology and governance,” he says. Mulder also predicts that the necessary integration of Gen-AI with other systems may be complex and time-consuming.
Yasar Goedecke, head of analytics and artificial intelligence (AI) at Lufthansa Technik (LHT), considers Gen-AI a highly disruptive technology, especially for MROs. LHT has already identified a double-digit number of use cases for Gen-AI in MRO.
To protect LHT’s intellectual edge, Goedecke will only mention a few possibilities. Short-term, he says Gen-AI can improve planning of maintenance events. “Gen-AI can assist our workers through a much more direct and efficient input of even unexpected findings,” he says. “For example, these could simply be voice-recorded into an AI device that directly connects the dots, understands the technical background and in turn automatically generates new work scopes and instructions.” When connected onward to tooling, parts or other downstream systems, Goedecke says this method could save time and costs, cushioning the effects unexpected findings have on turnaround times of almost every maintenance layover.
Longer term, Goedecke sees Gen-AI conserving knowledge. Unlike experienced mechanics, “AI never goes into retirement,” he says. It will be able to collect, retain and present interpretable and understandable knowledge, for example on factors that have led to repair decisions in the past that otherwise would get lost when experienced workers retire.
Still, there are hurdles to exploiting Gen-AI. Goedecke says the processes by which Gen-AI arrives at answers and recommendations are often not transparent. And Gen-AI may not be fully reliable in transferring knowledge from one problem to another. “Hence, potential tasks for Gen-AI still have to be defined with great accuracy and on a very small scale,” he says.
Nevertheless, for conventional office and administration tasks, LHT is already using Gen-AI-assisted automated translations of texts into various languages. LHT has also taken a few first steps into involving Gen-AI in certain creative processes, such as developing catchy text headlines, project acronyms or marketing mottos and slogans.
AAR Director of Strategy, Planning and Innovation Joel Blumenau is bullish on Gen-AI as well. “Whether we realize it or not, we are all using Gen-AI on a daily basis already to complete sentences in our emails,” he says.
Gen-AI is already embedded in AAR’s information security tools to better understand behaviors and threats and apply countermeasures. In the short term, Blumenau sees Gen-AI also helping mechanics access maintenance manuals. “For example, they could benefit from a hands-free, voice-activated interface with intelligent decision-making augmentation,” he says. AAR is talking to vendors about this sort of Gen-AI application. Gen-AI can also support intelligent chat-bots to exchange information with suppliers and customers.
Longer term, Blumenau sees no limit to Gen-AI’s impact on aviation and AAR. “Virtually any data-driven decision process could be automated, given availability of enough training data for the learning models,” he says. AAR is now looking at ways Gen-AI might optimize inventory management, predictive maintenance, warehouse operations, part trading and much more. Beyond this, AAR sees Gen-AI eventually aiding recruiting, procurement, sourcing and compliance with audits.
Blumenau believes the hardest implementation challenges will be people, not technology. Change management will be tough. And, to avoid biases in Gen-AI, AAR must use unbiased, real-world data sets to train the models
While global MROs with substantial IT departments have the resources to pursue these use cases, smaller MROs are not as advanced. For example, the Magnetic Group is still using AI only at a very basic level and has not yet looked at Gen-AI, according to a spokesperson.
However, Oliver Wyman Aviation Partner Derek Constanza sees Gen-AI spreading, “assisting with research that mechanics are forced to do today—for example, what do I do for this particular aircraft configuration, which parts are effective, troubleshooting, what is the best path and so forth.”
In a recent webinar, Oliver Wyman’s AI veterans gave all businesses, including but not limited to aircraft aftermarket companies, some tips on the new tools.
First, all companies will eventually make use of Gen-AI, the Wyman experts argued. Second, managers should beware of either overestimating Gen-AI’s short-term impact or underestimating its long-term impact. Third, current Gen-AI models are optimized for believability, not accuracy, which makes them useful for some purposes and less useful for others. Lastly, the experts note that there are many risks with using Gen-AI, but argue that these can be handled.
David Waller, a partner who leads Oliver Wyman’s data science and analytics practice, explained what is new about Gen-AI: inputs, outputs and “what goes on in the middle.”
Users can enter natural human language or pictures in Gen-AI. It can then generate essays or illustrations that feel human. In the middle of Gen-AI are models of “titanic size” that can, with easily accessible files of human knowledge, run regressions on a hundred billion parameters.
Gen-AI is thus capable of human-like creativity, but may be subject to human-like errors as well, so users must determine whether they value creativity over accuracy. However, Waller says experts can eventually “tame the models” to serve their purposes.
John Lester, partner in Wyman’s digital practice, believes the first business uses of Gen-AI will come as individual employees use it to be more productive in personal tasks such as fixing code, drafting an email or brainstorming a pitch to a client. He argues this is already happening and will extend to every organization in the world.
The next step might be companies using Gen-AI to draft routine documents, but companies must ensure the tool is sufficiently reliable. “If it works 80-90% of the time, is that enough?” asks Lester.
Gen-AI brings both old and new risks, according Sian Towson, an Oliver Wyman expert in AI risks. “It’s not optimized for accuracy,” he cautions. Instead, current Gen-AI models are optimized to appear human and be highly believable.
Gen-AI is not malicious itself, having no “intentionality,” Towson stresses. But, like the Internet—which, along with its massive benefits, allowed bad actors to scam and hack—Gen-AI gives bad actors powerful new tools for tricking and misleading. It can mimic the language, voice and signature of a real human being and create a deep-fake email. Regulations are thus needed, and companies will want these to be uniform across borders. Towson thinks Europe will lead in setting regulatory standards.
Despite risks, Lester warns that it would be a mistake for companies to avoid Gen-AI or prohibit employees from using it. “Let them use it and figure out safe ways to engage,” he says.
Then, Lester says major companies should start experimenting with developing their own Gen-AI models, using their own data to train the tool for their own purposes. “Don’t expect a clear, easy [return on investment] on your first proof of concept,” Lester says. “But you will learn a lot.”
Waller says one challenge is that it will be hard to change long-standing workflows to exploit Gen-AI capabilities, “but you have to wade into the waters.”
After all, Talia is eager for company.